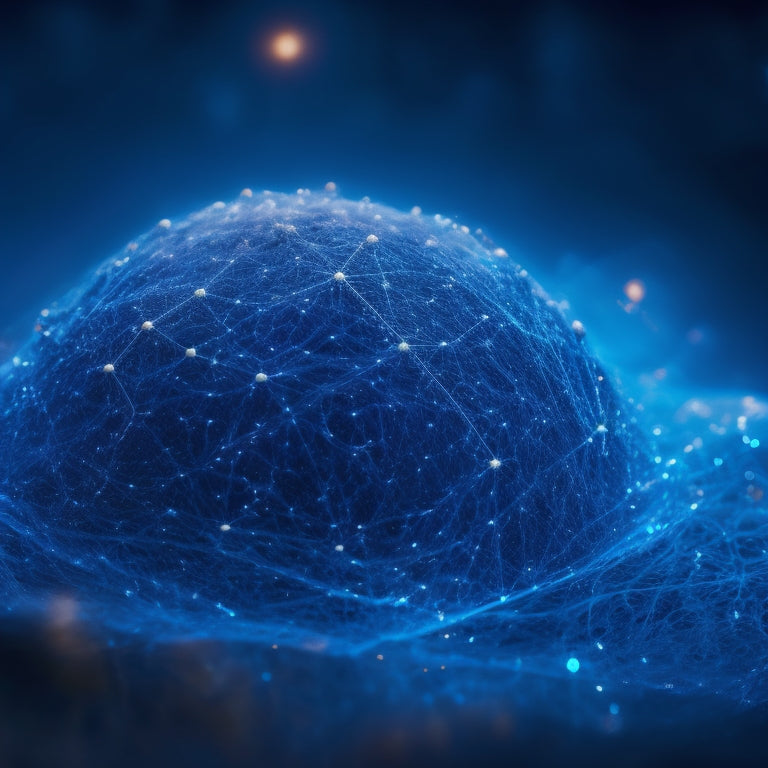
Revolutionary Deep Learning Unveiled for Online Knowledge
Share
Deep learning architectures have transformed online knowledge acquisition by automatically extracting insights from vast digital data, uncovering patterns and connections that may be missed by human analysts. These advanced models excel in analyzing complex patterns, particularly in natural language processing, enabling applications such as text classification, sentiment analysis, and language translation. By leveraging collaborative modeling and fine-tuning neural network architectures, deep learning enhances knowledge enhancement and enables personalized learning systems. As researchers continue to refine these architectures, the accuracy and potential of online knowledge analysis are poised to further accelerate, opening up new possibilities in the pursuit of knowledge.
Key Takeaways
• Deep learning excels in analyzing complex patterns, enhancing online knowledge acquisition with applications in text classification, sentiment analysis, and language translation.
• Sophisticated architectures, such as neural networks and transformer-based models, effectively process and extract insights from large data sets for thorough knowledge extraction.
• AI-powered tools integrated into collaborative learning environments improve interaction, engagement, and knowledge sharing among learners, enabling personalized learning experiences.
• Deep learning architectures automatically extract insights from vast digital data, uncovering patterns and connections that may be missed by human analysts, and enhancing information retrieval and decision-making.
• Future improvements in deep learning, such as refining prompt-engineering and expanding datasets, are crucial for developing more effective AI tools for online knowledge analysis.
Unleashing the Power of Deep Learning
Deep learning, a subset of machine learning, has emerged as a powerful tool for analyzing complex patterns in data, particularly in the domain of natural language processing. This technology has numerous applications, including text classification, sentiment analysis, and language translation.
In the context of online knowledge, deep learning applications can greatly enhance knowledge enhancement by automatically extracting relevant information from vast amounts of data. For instance, deep learning models can be trained to identify and categorize online learning resources, making it easier for learners to access and utilize relevant information.
Moreover, deep learning can facilitate the development of personalized learning systems, which can adapt to individual learners' needs and abilities. By harnessing the power of deep learning, online knowledge can be more effectively structured, accessed, and utilized.
Architectures for Online Knowledge Analysis
The development of sophisticated architectures is necessary to fully leverage the potential of deep learning in online knowledge analysis. It enables the creation of models that can effectively process and extract insights from large amounts of data.
Collaborative modeling approaches facilitate the integration of multiple data sources and perspectives, allowing for more thorough knowledge extraction.
Neural network architectures, such as feed-forward networks and transformer-based models, have shown promise in online knowledge analysis. These architectures can be fine-tuned for specific tasks, enabling the extraction of relevant knowledge from vast amounts of data.
Accuracy Results and Future Directions
Gunawardena et al.'s experiment yields accuracy results of 21.55% for the Doc2Vec feed-forward network and 43% for the fine-tuned large language model transformer, with the prompt-engineering approach achieving a notable 52.79% accuracy. These results demonstrate the potential of deep learning architectures in online knowledge analysis.
Future improvements can be made by expanding the dataset to include more diverse and thorough online knowledge sources. Additionally, refining the prompt-engineering approach can further enhance accuracy. These directions are essential for developing more effective AI tools that can efficiently analyze and provide insights from online knowledge.
Implications for Collaborative Learning Design
Online collaborative learning environments can greatly benefit from the integration of AI tools, leveraging the potential of deep learning architectures to facilitate more effective knowledge analysis and sharing.
This integration has significant design implications for collaborative learning environments, as AI-powered tools can enhance the interaction and engagement among learners.
Collaborative tools, such as discussion forums and group projects, can be augmented with AI-driven features that promote meaningful dialogue and knowledge construction.
The incorporation of AI tools can also enable personalized learning experiences, tailored to individual learners' needs and preferences.
Transforming Online Knowledge Acquisition
Deep learning architectures, particularly feed-forward networks and large language models, hold immense potential for revolutionizing online knowledge acquisition by automatically extracting insights and relationships from vast amounts of digital data.
This transformative technology enables the development of innovative applications that can efficiently process and analyze large datasets, uncovering patterns and connections that may have gone unnoticed by human analysts.
By leveraging these capabilities, online knowledge acquisition can be greatly enhanced, facilitating more accurate and efficient information retrieval, and ultimately leading to more informed decision-making.
As a result, the potential for deep learning to transform online knowledge acquisition is vast, and its implications for the future of online learning and education are profound.
Frequently Asked Questions
What Inspired the Creation of Gunawardena's Interaction Analysis Model?
Contrasting traditional pedagogical approaches, Gunawardena's Interaction Analysis Model (IAM) was inspired by the need to understand complex Social Dynamics and diverse Learning Paths in online educational settings, necessitating a nuanced framework for analyzing learner interactions.
Can Deep Learning Models Be Used for Real-Time Online Knowledge Analysis?
Yes, deep learning models can be used for real-time online knowledge analysis, providing instantaneous insights by leveraging knowledge graphs to represent complex relationships and facilitating the extraction of meaningful patterns from vast amounts of data.
How Do Doc2vec and BERT Architectures Differ in Natural Language Processing?
"In the real-time online knowledge analysis arena, Doc2Vec and BERT architectures diverge in their approach to natural language processing, with Doc2Vec utilizing fixed vector representations and BERT leveraging contextual embeddings and language representations for more nuanced understanding."
What Are the Potential Benefits of Integrating AI Tools in Online Learning Platforms?
Integrating AI tools in online learning platforms can facilitate personalized learning experiences and provide intelligent feedback, enabling tailored instruction, enhanced student engagement, and improved learning outcomes through adaptive assessments and real-time feedback.
Are There Any Ethical Concerns Related to Using AI in Online Knowledge Acquisition?
When integrating AI in online knowledge acquisition, ethical concerns arise, including AI accountability and exacerbating the digital divide, potentially perpetuating biases and unequal access, necessitating meticulous consideration of these issues to guarantee equitable and responsible AI implementation.
Related Posts
-
Drawer Inserts for Modern Bathroom Aesthetics
You invest in a modern bathroom aesthetic, but cluttered drawers and disorganized storage can quickly undermine the s...
-
Boost Your Grades With Proper Citations
Proper citation is an important aspect of academic writing that can greatly impact the credibility and validity of re...
-
Mastering Project Management Organization for Success
You know that mastering project management organization is essential for success, but it requires a strategic approac...